Solid form selection is a crucial part of active pharmaceutical ingredients (API) development with over 90 % of API’s on the market approved as solid formulations. Each solid form can have significantly different physicochemical properties therefore it is important to select an appropriate solid form that optimizes the properties, ensuring the API will be effective in the body and that it is cost effective to produce. Additionally, different solid forms can have different relative stabilities, therefore it is necessary to understand the solid form landscape in order to avoid unwanted forms with different properties appearing during manufacturing, storage or transport, furthermore this will help avoid undermining of an API’s legal protection from generics.
Outlined below is a method for screening utilizing stat-of-the-art artificial intelligence to predict co-crystal formation based on a machine learning link prediction algorithm to identify suitable conformers. These identified matches are then tested using the Crystal16, which provides a medium throughput platform for co-crystal screening.
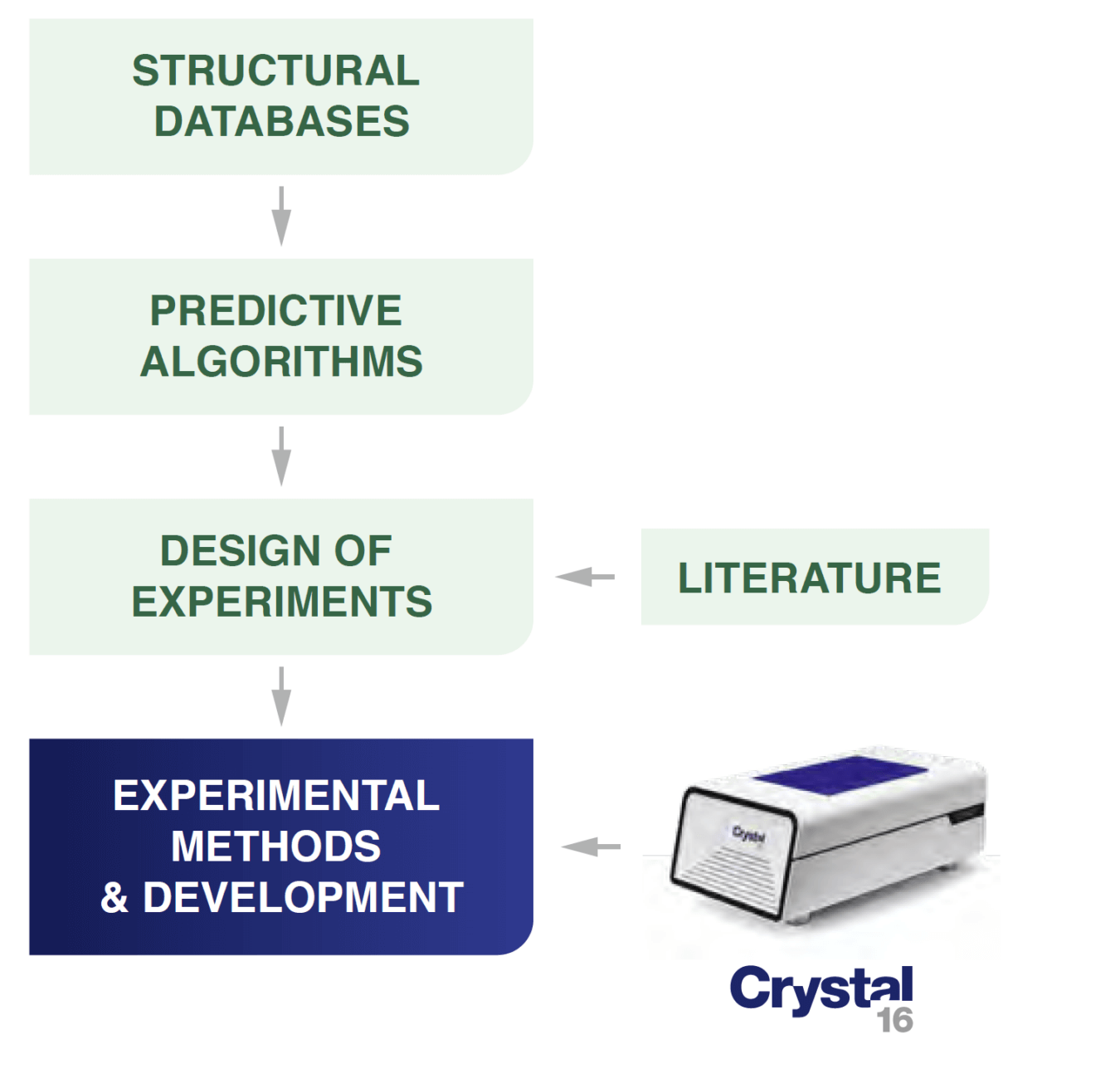
Figure 1: Workflow for co-crystal screening using AI and Crystal16
Solid form and co-crystals
There are several types of solid forms available to scientists when selecting which solid form they want to take for formulation development. Amorphous solids have no long range order and have a high energy state, this allows them to have high solubility but also unstable. Different packing patterns of the same molecule in a crystal lattice results in different polymorphs of the API. Secondary molecules can be incorporated into the crystal lattice. When solvent or water is incorporated in the crystal structure they are classified as solvates or hydrates respectively. When a secondary molecule ionically bonded to the API then a salt is formed. If this secondary molecule is hydrogen bonded to the API then a co-crystal is formed.
Co-crystals are an area gaining growing interest due to their ability to modify properties depending on the co-former used. Taste of an API can be modified using artificial sweeteners, permeability can be improved by using lipophilic co-formers, and melting point can be improved using molecules with high melting points.
Screening with AI
Over the years, several computational methods have been developed for ascertaining and ranking the probability of co-crystal formation of API-co-former pairs. Some of the more accurate prediction techniques are based on experimental crystallographic data, usually from the Cambridge Structural Database (CSD) which curates the information of over 1 million crystal structures determined at Å resolution. A new approach for co-crystal prediction was recently reported and is based on a machine learning link prediction algorithm. On a highly simplistic level, this AI method works as follows:
- All co-crystal structures from the CSD are collected
- The relation between APIs and co-formers are mapped as an interconnected network (social network analogy)
- The resulting correlation network is analyzed for missing connections between nodes, more specifically between the API and coformers for similar molecules
This new workflow was demonstrated using Febuxostat which is used for the prevention of gout by decreasing the amount of uric acid the body produces. It was chosen as the test API due to its very rich, but not fully explored solid form landscape, including several known polymorphs, solvates and co-crystals.[i],[ii]
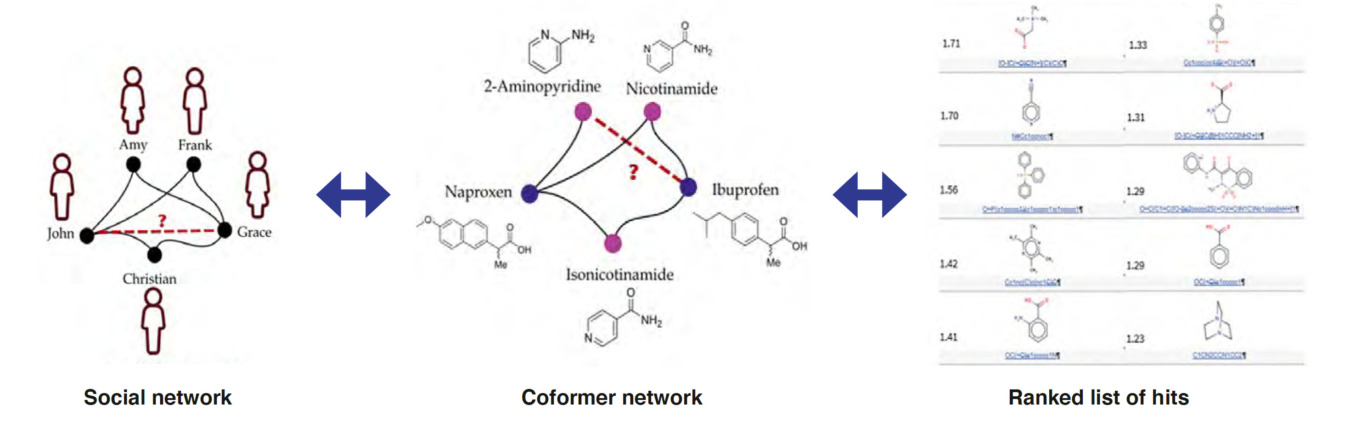
Figure 2: Schematic representation of the link prediction algorithm and ranking of some potential co-formers for febuxostat co-crystals
Experimental
Using the machine learning p-toluenesulfonic acid was selected as a co-former likely to form a co-crystal. Experiment co-crystal screening implies testing several crystallization methods, API and co-former concentrations, in various solvents and thermodynamic conditions. Computational methods can point you towards a likely co-former, and therefore significantly reduce the number of experiments required for a successful hit. A wide range of solvents can further complicate things, through the formation of solvates. For this reason, 4 solvents were chosen for this study, due to their low likelihood of forming solvates and for their good solvation ability towards both API and co-former. Having reduced the complexity of the screening as much as possible, there is still the need to test several crystallization modes, as well as concentrations. The Crystal16 device is ideally suited for such work and is also a well-known research tool used for co-crystal screening.[i],[ii]
Conclusions
The current case study showed that an effective co-crystal screening protocol can be defined using machine learning to identify suitable co-formers and Crystal16 instrument to experimentally find them. The in-situ light transmission measurement during the co-crystal screen offers an important advantage for the scale-up and process development design, by allowing the determination of the solubility curve and MSZW for the co-crystal:solvent system. Using the Crystal16, within 1 day you can test one conformer in 4 solvents simultaneously, with 4 concentrations in each solvent and maximize co-crystal formation by fully exploring the concentration range. In one day, you can reliably test co-crystal formation for a high ranked co-former and get information on its crystallization conditions for further scale-up and process development.
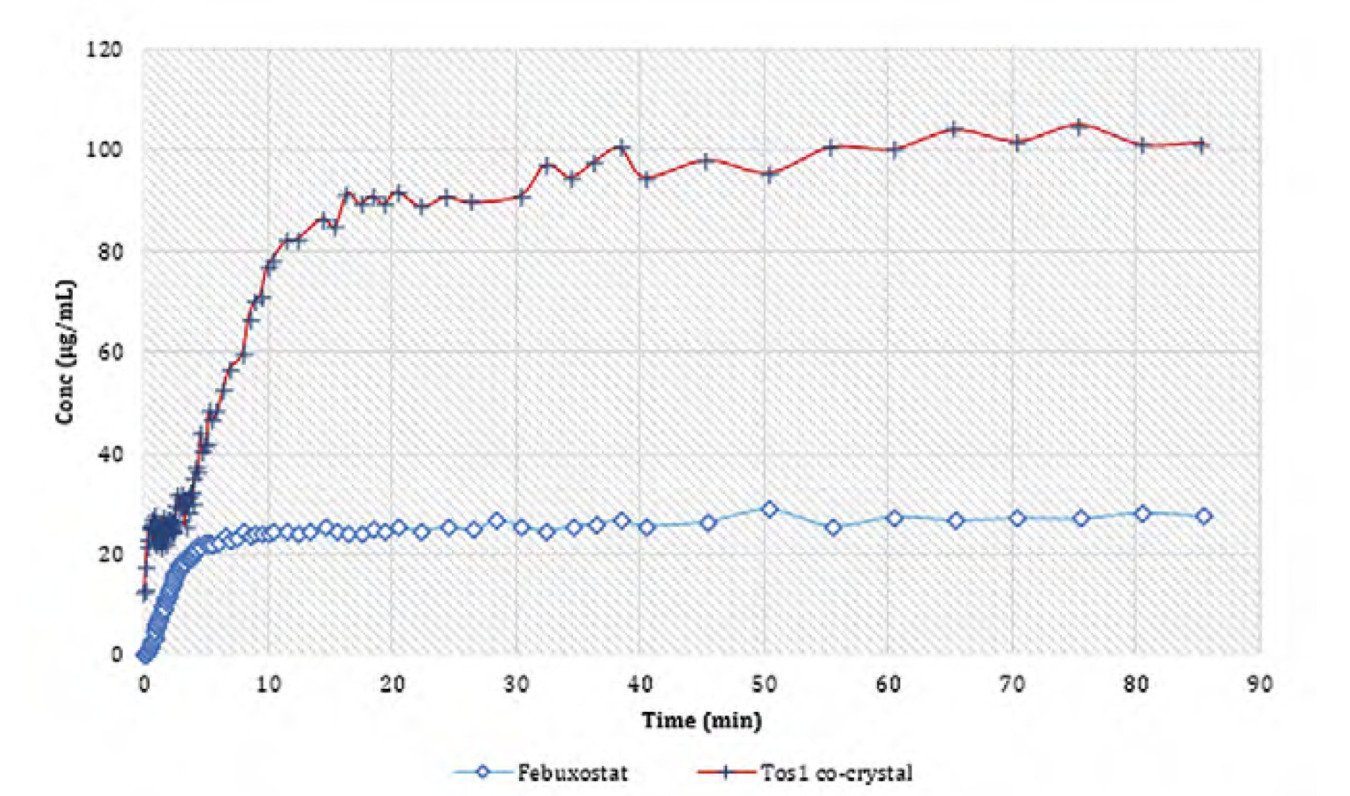
Figure 3: The improved dissolution curves of the Tos1 co-crystal and the starting material (FEB) in water.
Acknowledgements
Technobis Crystallization Systems is deeply grateful to Dr. Mihaela Pop and Dr. Doriana Ungur from TeraCrystal for their help with this case study. The experimental work was performed in the laboratories of TeraCrystal. The AI calculations were performed at Radboud University Nijmegen and were presented with the help of Dr. Rene de Gelder and Dr. Jan Joris Devogelaer.
[i] Maddileti, D.; Jayabun, S. K.; Nangia, A. Crystal Growth& Design, 2013, 13 (7), 3188-3196 https://doi.org/10.1021/cg400583z
[ii] Patel, J., Jagia, M., Bansal, A.K.; Patel, S. J. Pharm. Sci., 2015, 104: 3722-3730. https://doi.org/10.1002/jps.24570
[iii] a) ter Horst, J. H.; Deij, M. A.; Cains, P. W. Crystal Growth&Design 2009, 9,
1531−1537 https://doi.org/10.1021/cg801200h
[iv] Li, W.; de Groen, M.; Kramer, H.JM.; de Gelder, R.; Tinnemans, P.; Meekes, H.; ter Horst, J.H. Crystal Growth&Design 2021 21 (1), 112-124 https://doi.org/10.1021/acs.cgd.0c00890
Curious to learn more?
Read more about the experiments in this article in the application note "Co-crystal Screening: from Smart Design to Effective Experimentation".